The HR tech market is big and growing and shows no sign of slowing down. HR cloud solutions alone were a $148 billion market in 2019, and a survey of HR leaders from PwC found that 74% plan to increase HR tech spending in 2020. Their top concerns were attracting and keeping top talent and helping employees reach their potential. In response, there’s been an explosion of analytics and coaching platforms that give users the ability to passively understand information about digital relationships at work by analyzing digital communications like e-mails, chat messages, and calendars.
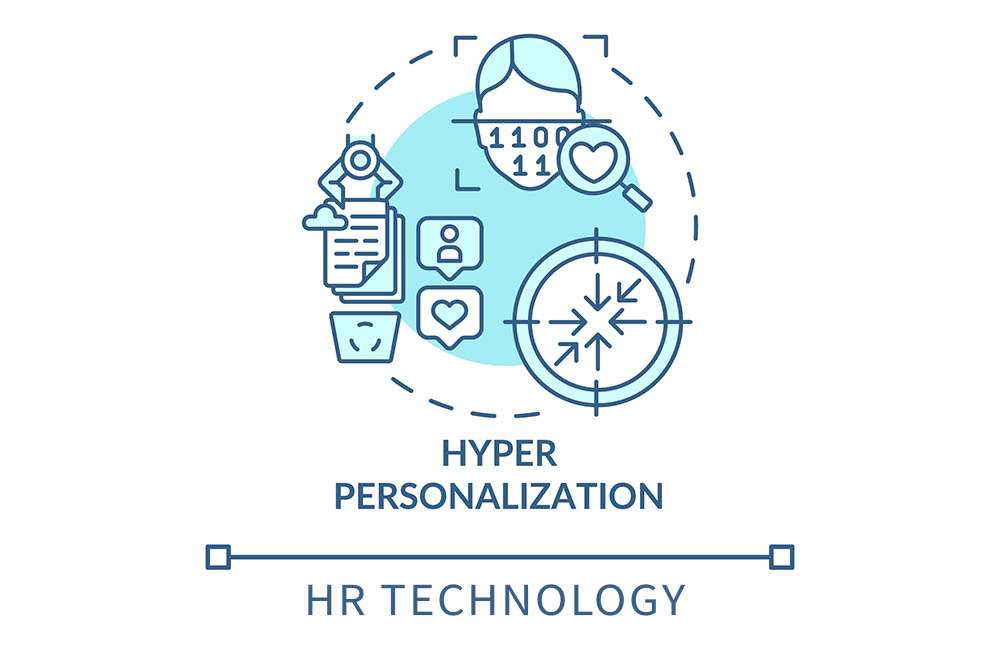
These platforms fall into one of two categories based on the type of information they ingest. Some measure metadata (or “digital exhaust”), while others use machine learning models to analyze the actual content of messages.
At the same time, HR tech is becoming hyperpersonalized. New HR tools seek to personalize how individuals receive workplace training, how companies hire new employees, or how they match existing employees with new career opportunities. These technologies can increase employee engagement by making the hiring, training/learning, and advancement process correspond to a specific employee’s interests and strengths. This can have major benefits for a business: Companies with a high level of engagement report 22% higher productivity, according to Gallup data, and the number one reason employees leave jobs is they’ve stopped growing. Hyperpersonalization can address these issues.
Going back to my first point, the question becomes: Which of these two types of analytics and coaching platforms (metadata or message content analysis) is more personalized? While measuring metadata produces some useful information, I believe measuring the intent of a message’s content creates more effective coaching and analytics tools overall. Here’s how these two processes work and why I believe content analysis is the more effective option to achieve this goal of hyperpersonalization.
Data About Data
You can think of metadata as data about other data. So, for an e-mail, for example, the metadata would include the sender of the e-mail, any recipients, and the time the e-mail was sent. With that information, an HR platform can know if people are chatting after hours, who is initiating messages with whom, and how often two people are communicating. But metadata do not capture what is happening during the conversation—just that the conversation happened. Tools that rely on metadata can measure things like how many one-on-one messages someone has, how often someone sends messages after work hours, and how frequently someone communicates with his or her team. That’s valuable information, but ultimately, these tools can’t tell users anything about the content of messages, so there’s a limit to what they can provide.
Other tools use machine learning and natural language processing models to analyze the content of messages and determine what the intent and tone of the messages are. This allows them to measure things like responsiveness, politeness, asking for opinions, giving recognition, and requesting feedback, which cannot be gathered from metadata alone. This analysis can lead to more actionable insights that help users achieve a better understanding of their relationships with their team members (i.e., do they struggle with giving their team enough recognition, or does it take them a long time to respond to messages?). This analysis can also target specific behaviors that are known to have positive downstream effects—aka the “soft skills” of leadership. Finally, harnessing message content can provide a richer understanding of company culture than organizational network analysis based on metadata alone can. Let’s walk through each of these differences in more detail.
Actionable Insights to Help People Leaders
Self-awareness is a critically important tool for managers, correlating highly with managerial performance, leader effectiveness, and report satisfaction. HR tools that can analyze message content can help users develop self-awareness by showing them their bad habits. Metadata analysis can reveal some of these habits, like sending too many messages after hours. But others, like asking teams for their opinions and responding to their input thoughtfully, require content analysis to surface. Furthermore, inclusion, a state in which every employee feels valued and respected and has equal opportunities within the organization, cannot be achieved without managerial self-awareness. For example, a manager may not be aware that he or she asks for opinions more often from male employees than from female employees. This behavior precludes an inclusive environment, but it cannot be changed without knowledge of the bias.
Developing Soft Skills
The ability to measure message content can detect and encourage leadership behaviors known to have positive downstream effects on employees. For instance, behaviors like expressing doubt, requesting feedback, and sharing opinions promote psychological safety in teams. This concept, which comes from the work of Harvard Professor Amy Edmondson, means that members feel that the team is a safe environment to take interpersonal risks.
In 2012, Google found that psychological safety was the most important feature of high-performing teams. Message content analysis can show leaders when they engage in behaviors that will promote psychological safety (or another desirable characteristic, like empathy) and encourage them to do it more often. This will make their teams more effective in the long run. Picking out these behaviors requires a level of nuance that metadata analysis cannot provide.
Company Culture
Sameer B. Srivastava, Associate Professor at UC Berkeley’s Haas School of Business, published a paper in 2017 analyzing electronic message content (instead of only metadata) captured over 5 years at a company of around 600 employees. His analysis looked at organizational enculturation, or “culture fit,” over time based on how employees adapted and conformed to their peers’ speaking habits. Looking at the intent of messages allows teams to build models like this that show a company’s overall culture. For example, one company might value positivity and encouragement but have managers who struggle to unplug and often work at all hours.
This company would have a higher percentage of “giving recognition” messages and a higher percentage of messages sent after hours. Another company might score low in these categories but have a more collaborative culture that’s reflected in a high percentage of messages that share information and ask for feedback. Many HR and people analytics teams would find this type of analysis valuable, but it can’t be done without detailed message content analysis.
As HR tech continues to grow and more solutions hit the market, remember that not all of them work the same “under the hood,” and organizations should select the options that best fit the type of analysis they need to perform. What’s most exciting to me is that this field is still young, and our ability to perform this analysis is still developing! Imagine a future in which managers could get real-time prompts to recognize employees after they report they’ve completed a task in Slack or a “to-do” list that fills up automatically as the user makes commitments in an e-mail. What other ways might data be used to make an employee’s experience smoother and more productive?
Joseph Freed is Cofounder and CEO at digital leadership platform Cultivate. Freed has worked at or led several start-ups focused on online learning and HR technology, has an MBA from the New York University, and lectures at UC Berkeley Extension. Cultivate recently raised an $8 million funding round.